새 문서: 분류: USENIX FAST Ruoyu Qin, Zheming Li, Weiran He, Jialei Cui, Feng Ren, Mingxing Zhang, Yongwei Wu, Weimin Zheng, Xinran Xu USENIX FAST 2025 == 개요 == == Motivation & Importance == == Challenge == == Background == == Main Idea == == Design == == Result == == Contribution == == Implementation == |
편집 요약 없음 |
||
(같은 사용자의 중간 판 하나는 보이지 않습니다) | |||
1번째 줄: | 1번째 줄: | ||
[[분류: USENIX FAST]] | [[분류: USENIX FAST]] | ||
MOONCAKE: Trading More Storage for Less Computation — A KVCache-centric Architecture for Serving LLM Chatbot | |||
Ruoyu Qin, Zheming Li, Weiran He, Jialei Cui, Feng Ren, Mingxing Zhang, Yongwei Wu, Weimin Zheng, Xinran Xu | |||
USENIX FAST 2025 | |||
== 개요 == | == 개요 == | ||
MOONCAKE는 Moonshot AI의 LLM 챗봇 서비스인 Kimi의 서빙 플랫폼으로, KVCache 중심의 분리형(disaggregated) 아키텍처를 도입하여 스토리지를 적극적으로 활용해 연산을 줄이는 구조를 제안한다. CPU, DRAM, SSD, NIC 등의 자원을 GPU와 독립적으로 활용하여 분산된 KVCache 시스템을 구성하고, 이를 통해 SLO([[TTFT]]-Time to First Token, [[TBT]]-Time Between Tokens)를 만족하면서도 처리량을 대폭 향상시켰다. | |||
== Motivation & Importance == | == Motivation & Importance == | ||
LLM 서비스는 각 요청의 길이, 도착 시간, SLO 등 다양한 제약을 가지며, 고정된 GPU 자원 내에서 최대한 많은 요청을 처리해야 한다. | |||
이때, TTFT와 TBT는 서로 Trade-off관계에 있으며, KVCache의 Scheduling에 영향을 받는다. MOONCAKE는 이러한 상황에서 GPU 자원을 효율적으로 활용하고, 자주 사용되는 KVCache를 재활용함으로써 연산량을 줄이고 처리량을 높이는 것이 목적이다. | |||
* Goal 1: Transfer as mush reusable KVCache as possible to decoding node from prefill node | |||
** Large-capacity KVCache storage | |||
** Low-latency and high-bandwidth KVCache transfer | |||
** KVCache-aware scheduling | |||
* Goal 2: Continuously stream the output KVCache to the corresponding decoding instance | |||
** Disaggregate the prefill and decoding nodes for parallelism | |||
** Group the CPU, DRAM, SSD and RDMA resources of the GPU | |||
* Goal 3: Load the KVCache and add the request to the continuous batching process at the decoding instance for generating request outputs | |||
** Global scheduler named Conductor | |||
== Challenge == | == Challenge == | ||
* Prefill과 Decoding 스테이지의 이질성 | |||
* Prefix 재활용에 따른 복잡한 캐시 스케줄링 | |||
* 고대역폭 네트워크 구축 | |||
== Main Idea == | == Main Idea == | ||
"More Storage for Less Computation" 전략을 통해 이전 요청에서 생성한 KVCache를 prefix match를 통해 재사용함으로써 prefill 단계의 연산을 줄이고 응답 속도를 높인다. 이를 위해: | |||
* 분산 KVCache 풀(Mooncake Store)을 구성 | |||
* prefix match 기반의 캐시 중심 스케줄링 도입 | |||
* 높은 대역폭의 RDMA 네트워크 기반 전송 엔진 설계 | |||
== Design == | == Design == | ||
[[파일:USENIX FAST 2025 MoonCacke.png|섬네일]] | |||
* 아키텍처 중심: Prefill과 Decoding 노드를 분리하고, CPU/DRAM/SSD를 조합해 MOONCAKE Store를 구성. Hashed-key를 통해서 효율적인 Key search가 가능하게 함. | |||
* 스케줄러(Conductor): 요청을 prefix hit 정도, 부하 상태, 대기 시간 등을 종합 고려하여 최적의 prefill/decoding 인스턴스를 선택. '''Load뿐만 아니라 Prefix cache hit length까지 고려'''한다는 것이 이 논문의 핵심. 스케쥴러의 Policy는 Offline-data로 학습된 Polynomial regression model을 이용하여 구현. | |||
* Chunked pipeline parallelism (CPP): 긴 컨텍스트 처리 시 prefill을 chunk 단위로 병렬 실행하여 TTFT 단축. | |||
* Cache Load Balancing: Frequent access cache - Distribute across the nodes, Cache used by a single application - Do not distribute across multiple nodes | |||
** Unlike the prefill time, workloads are highly dynamic and change significantly over time | |||
** Heuristic-based automated hotspot migration scheme | |||
** Heuristic 1: If an instance is busy, migrate KVCache to un-busy instance | |||
** Heuristic 2: Multiply threshold to the local KVCache, rather than choosing best-match prefix length node | |||
== Contribution == | == Contribution == | ||
# KVCache 중심의 분리형 LLM 서빙 아키텍처 제안 | |||
# 대용량 분산 KVCache 풀(Mooncake Store)의 설계 및 구현 | |||
# 캐시 인식 스케줄링 알고리즘 도입 | |||
# 실 서비스(Kimi)에서의 검증 및 오픈소스 제공 |
2025년 4월 10일 (목) 12:47 기준 최신판
MOONCAKE: Trading More Storage for Less Computation — A KVCache-centric Architecture for Serving LLM Chatbot
Ruoyu Qin, Zheming Li, Weiran He, Jialei Cui, Feng Ren, Mingxing Zhang, Yongwei Wu, Weimin Zheng, Xinran Xu USENIX FAST 2025
개요
MOONCAKE는 Moonshot AI의 LLM 챗봇 서비스인 Kimi의 서빙 플랫폼으로, KVCache 중심의 분리형(disaggregated) 아키텍처를 도입하여 스토리지를 적극적으로 활용해 연산을 줄이는 구조를 제안한다. CPU, DRAM, SSD, NIC 등의 자원을 GPU와 독립적으로 활용하여 분산된 KVCache 시스템을 구성하고, 이를 통해 SLO(TTFT-Time to First Token, TBT-Time Between Tokens)를 만족하면서도 처리량을 대폭 향상시켰다.
Motivation & Importance
LLM 서비스는 각 요청의 길이, 도착 시간, SLO 등 다양한 제약을 가지며, 고정된 GPU 자원 내에서 최대한 많은 요청을 처리해야 한다.
이때, TTFT와 TBT는 서로 Trade-off관계에 있으며, KVCache의 Scheduling에 영향을 받는다. MOONCAKE는 이러한 상황에서 GPU 자원을 효율적으로 활용하고, 자주 사용되는 KVCache를 재활용함으로써 연산량을 줄이고 처리량을 높이는 것이 목적이다.
- Goal 1: Transfer as mush reusable KVCache as possible to decoding node from prefill node
- Large-capacity KVCache storage
- Low-latency and high-bandwidth KVCache transfer
- KVCache-aware scheduling
- Goal 2: Continuously stream the output KVCache to the corresponding decoding instance
- Disaggregate the prefill and decoding nodes for parallelism
- Group the CPU, DRAM, SSD and RDMA resources of the GPU
- Goal 3: Load the KVCache and add the request to the continuous batching process at the decoding instance for generating request outputs
- Global scheduler named Conductor
Challenge
- Prefill과 Decoding 스테이지의 이질성
- Prefix 재활용에 따른 복잡한 캐시 스케줄링
- 고대역폭 네트워크 구축
Main Idea
"More Storage for Less Computation" 전략을 통해 이전 요청에서 생성한 KVCache를 prefix match를 통해 재사용함으로써 prefill 단계의 연산을 줄이고 응답 속도를 높인다. 이를 위해:
- 분산 KVCache 풀(Mooncake Store)을 구성
- prefix match 기반의 캐시 중심 스케줄링 도입
- 높은 대역폭의 RDMA 네트워크 기반 전송 엔진 설계
Design
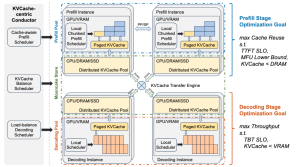
- 아키텍처 중심: Prefill과 Decoding 노드를 분리하고, CPU/DRAM/SSD를 조합해 MOONCAKE Store를 구성. Hashed-key를 통해서 효율적인 Key search가 가능하게 함.
- 스케줄러(Conductor): 요청을 prefix hit 정도, 부하 상태, 대기 시간 등을 종합 고려하여 최적의 prefill/decoding 인스턴스를 선택. Load뿐만 아니라 Prefix cache hit length까지 고려한다는 것이 이 논문의 핵심. 스케쥴러의 Policy는 Offline-data로 학습된 Polynomial regression model을 이용하여 구현.
- Chunked pipeline parallelism (CPP): 긴 컨텍스트 처리 시 prefill을 chunk 단위로 병렬 실행하여 TTFT 단축.
- Cache Load Balancing: Frequent access cache - Distribute across the nodes, Cache used by a single application - Do not distribute across multiple nodes
- Unlike the prefill time, workloads are highly dynamic and change significantly over time
- Heuristic-based automated hotspot migration scheme
- Heuristic 1: If an instance is busy, migrate KVCache to un-busy instance
- Heuristic 2: Multiply threshold to the local KVCache, rather than choosing best-match prefix length node
Contribution
- KVCache 중심의 분리형 LLM 서빙 아키텍처 제안
- 대용량 분산 KVCache 풀(Mooncake Store)의 설계 및 구현
- 캐시 인식 스케줄링 알고리즘 도입
- 실 서비스(Kimi)에서의 검증 및 오픈소스 제공